Agenda
MSc SS Thesis Presentation
- Friday, 3 December 2021
- 15:00-15:35
Analyzing Functional Ultrasound Images of the Brain Using Tensor Decompositions
Arda Kaygan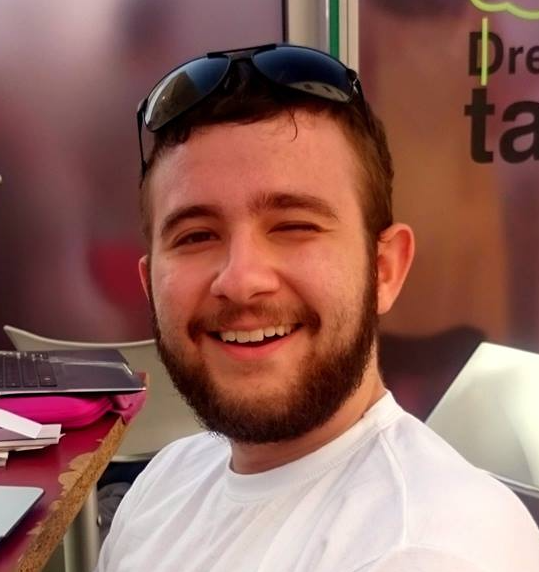
Functional ultrasound (fUS) is a neuroimaging modality that offers high spatial and temporal resolution while also providing portability. In this thesis, neuroimaging data acquired with fUS at Center for Ultrasound and Brain imaging at Erasmus MC (CUBE) is processed. Due to the fact that fUS data is inherently multidimensional, we propose using tensor decompositions, tensors here referring to generalizations of matrices, for processing of fUS data.
We define two main research questions regarding fUS data analysis. First, for compressing the large-scale raw beamformed fUS data, we apply sequentially truncated multilinear singular value decomposition. This compression method is compared against ensemble averaging used in the conventional pipeline, and shown to provide a higher compression rate while preserving more temporal resolution for specific ranks. Furthermore, it is observed to denoise the data, resulting in a more precise extraction of the active region of Superior Colliculus using correlation maps.
Secondly, in order to investigate the advantage of multi-slice processing that incorporates 3-D information, blind-source separation methods are applied to single slice and two-slice fUS recordings. After applying independent component analysis (ICA) to the matricized data as a benchmark method, block termdecomposition (BTD) is used as a way of processing the data as it is, in its natural 3-D structure without vectorization. Through a simulation study, it is shown that the method is able to separate two images even when using a rank that is lower than the true rank, as well as in noisy conditions. Subsequently, BTD is applied to real 4-D fUS data formed by concatenation of slices in a new dimension. However, this method is seen to perform worse than single slice ICA in terms of extracting the active regions. In order to amplify common information between slices, a new 3-D data structure is then formed by summing the fUS data of two slices. For extraction of this common information, a BTD is then applied to the aggregate 3-D data. The findings of this decomposition reveal that both taking a longer portion of single slice data and incorporating the second slice helps to achieve better results.
Additional information ...Agenda
- Tue, 30 Apr 2024
- 10:00
- HB18.090
MSc SPS Thesis presentation
Wim Kok
A SystemC SNN model for power trace generation
- Mon, 6 May 2024
- 12:30
- Aula Senaatszaal
PhD Thesis Defence
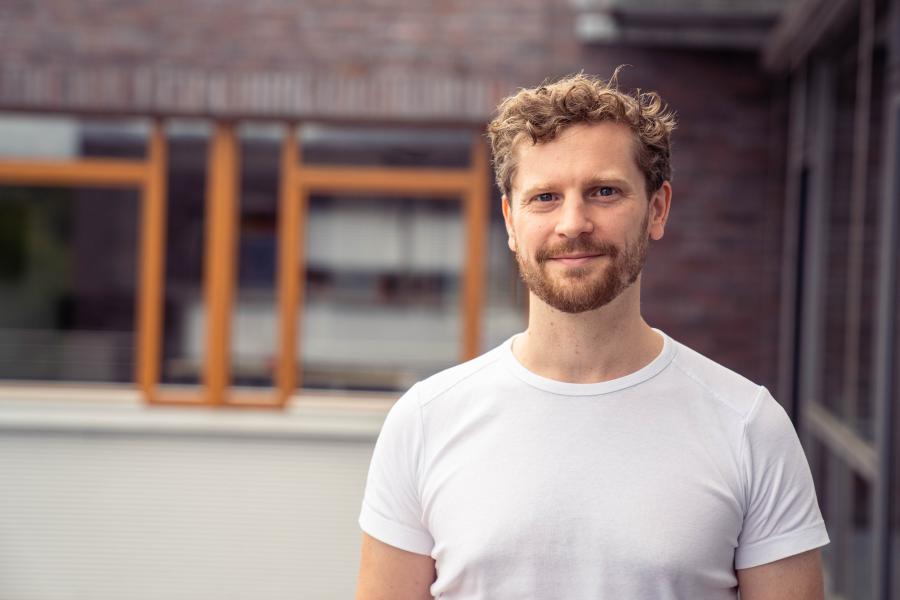
Christoph Manss
Multi-agent exploration under sparsity constraints
- Tue, 21 May 2024
- 10:00
- Aula Senaatszaal
PhD Thesis Defence
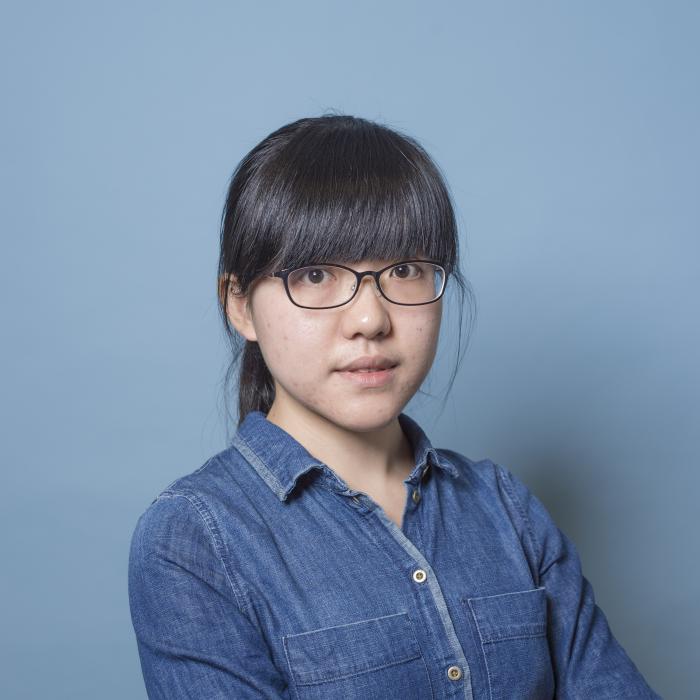
Wangyang Yu
- 27 -- 28 May 2024
- Aula, TU Delft
Conferences

44th Benelux Symposium on Information Theory and Signal Processing (SITB'24, Delft)
- Tue, 18 Jun 2024
- 15:00
- Aula Senaatszaal
PhD Thesis Defence
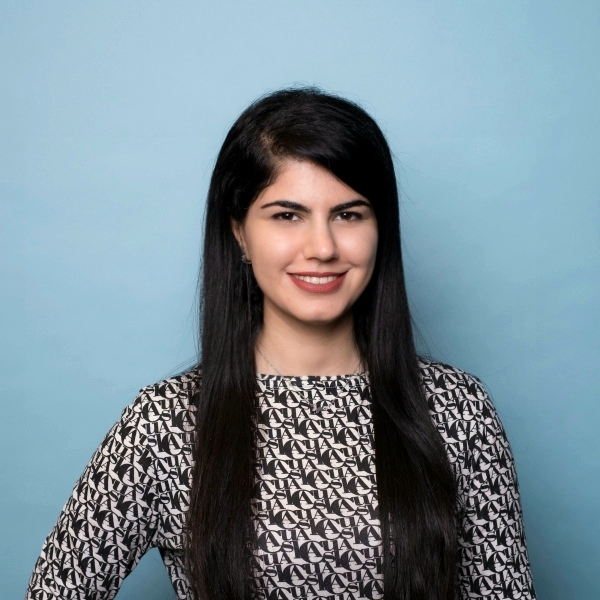
Hanie Moghaddasi
Model-based feature engineering of atrial fibrillation
- Mon, 24 Jun 2024
- Aula, TU Delft
Conferences
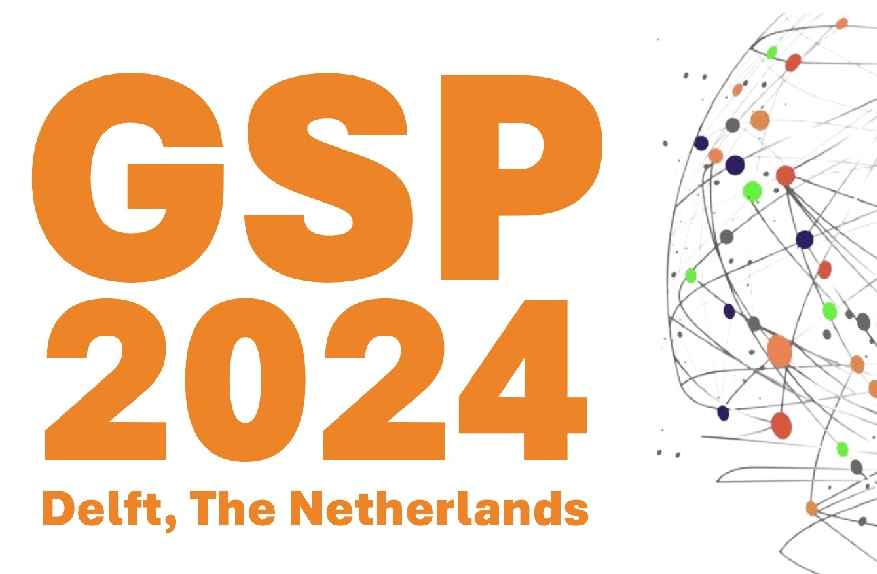