Agenda
Signal Processing Seminar
- Thursday, 12 April 2018
- 13:00-15:00
- HB 17.150
Compressive Covariance Sensing
Geert Leus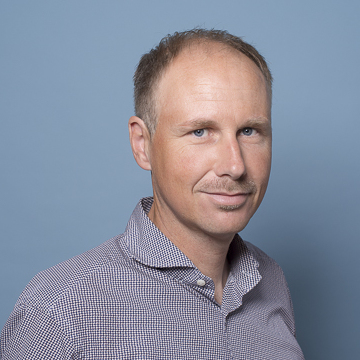
There are many engineering applications that rely on frequency or angular spectrum sensing, such as cognitive radio, radio astronomy, radar, seismic acquisition, and so on. Many of these applications do not require the reconstruction of the full signal, and can perfectly rely on an estimate of the power spectral density (PSD), or in other words, the second-order statistics of the signal. However, the large bandwidths of the involved signals lead to high sampling rates and thus high sampling costs, which can be prevented by a direct compression step carried out in the analog domain (e.g., by means of an analog-to-information converter, multi-coset sampling, analog beamforming, antenna selection, etc.). This leads to the problem of sensing the PSD or covariance using compressive observations, labeled as compressive covariance sensing (CCS). In this tutorial we will give an overview of the state-of-the-art in CCS and present its connections to compressive sensing (CS). We focus on the design constraints of the compression matrices, which are completely different as in classical CS, and elaborate on the estimation/detection techniques to sense the covariance using compressive measurements. In this context, both non-uniform and random sampling are discussed. We further elaborate on distributed CCS, where compressive measurements in one domain are fused in the dual domain, i.e., temporal compressive measurements are gathered at different spatial sensors or spatial compressive measurements from different time slots are combined. Finally, connections to super resolution techniques such as atomic norm minimization are discussed. We end this tutorial by sketching some open issues and presenting the concluding remarks.
Additional information ...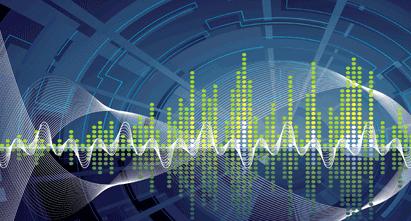
Agenda
- Tue, 30 Apr 2024
- 10:00
- HB18.090
MSc SPS Thesis presentation
Wim Kok
A SystemC SNN model for power trace generation
- Mon, 6 May 2024
- 12:30
- Aula Senaatszaal
PhD Thesis Defence
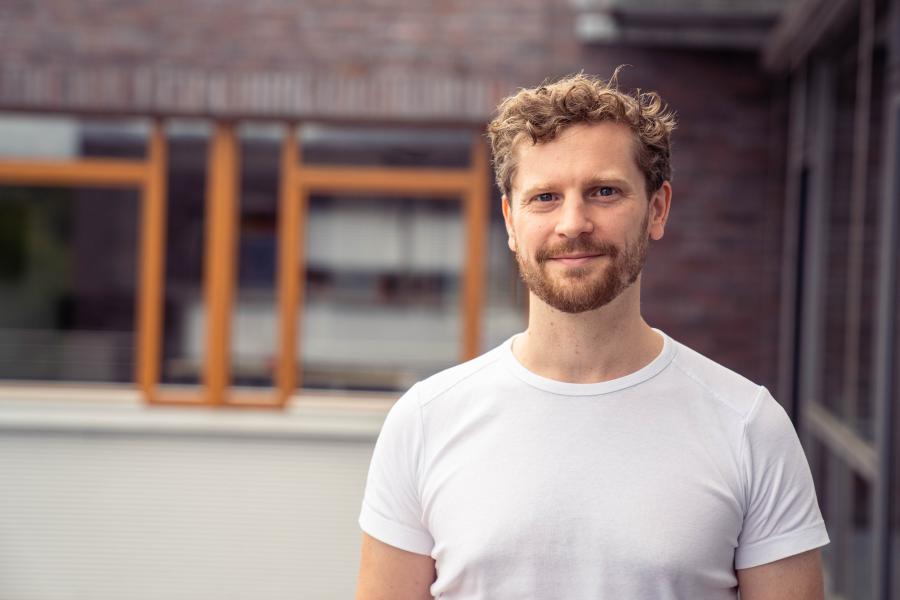
Christoph Manss
Multi-agent exploration under sparsity constraints
- Tue, 21 May 2024
- 10:00
- Aula Senaatszaal
PhD Thesis Defence
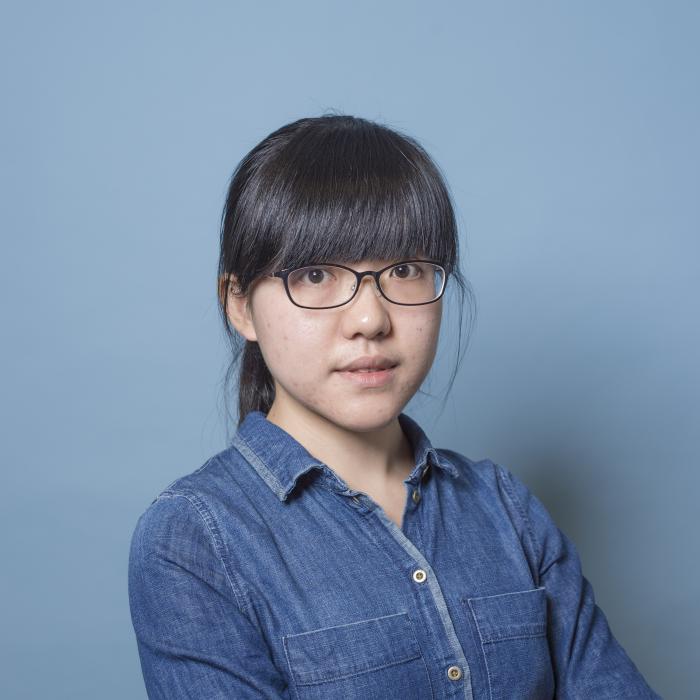
Wangyang Yu
- 27 -- 28 May 2024
- Aula, TU Delft
Conferences

44th Benelux Symposium on Information Theory and Signal Processing (SITB'24, Delft)
- Tue, 18 Jun 2024
- 15:00
- Aula Senaatszaal
PhD Thesis Defence
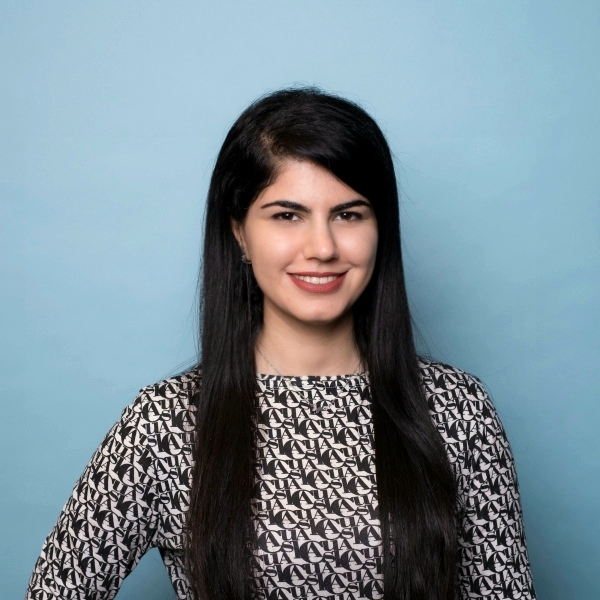
Hanie Moghaddasi
Model-based feature engineering of atrial fibrillation
- Mon, 24 Jun 2024
- Aula, TU Delft
Conferences
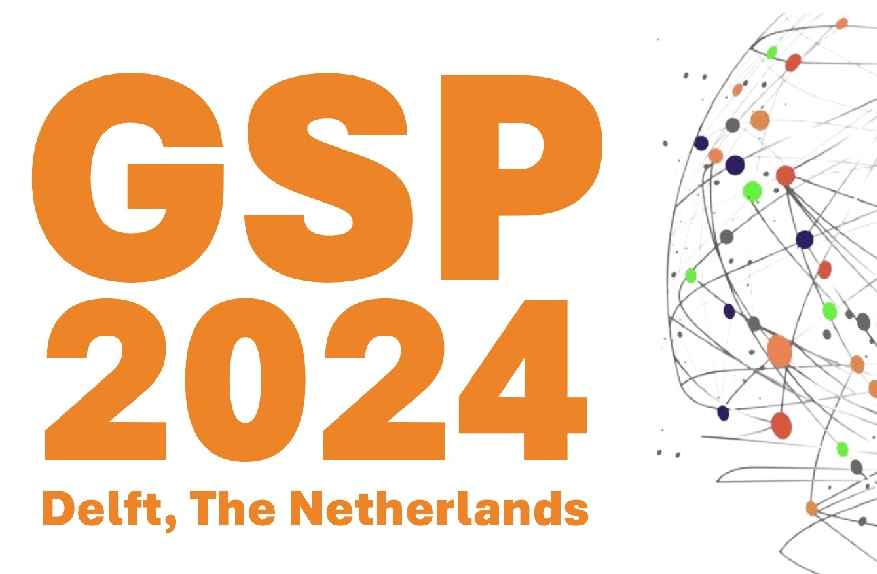